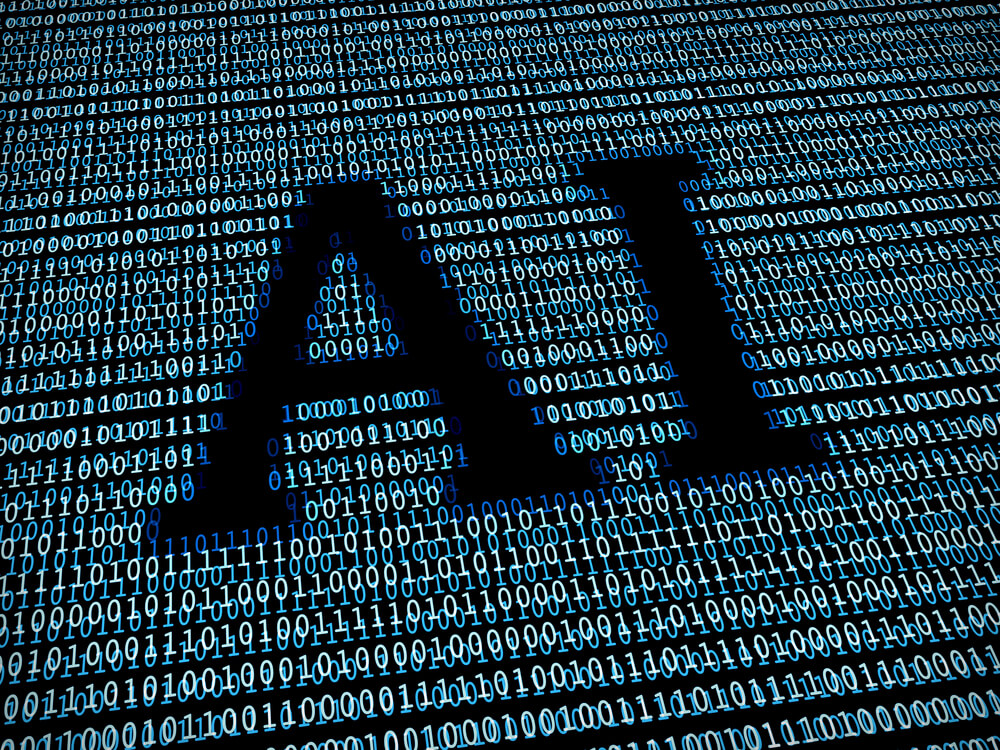
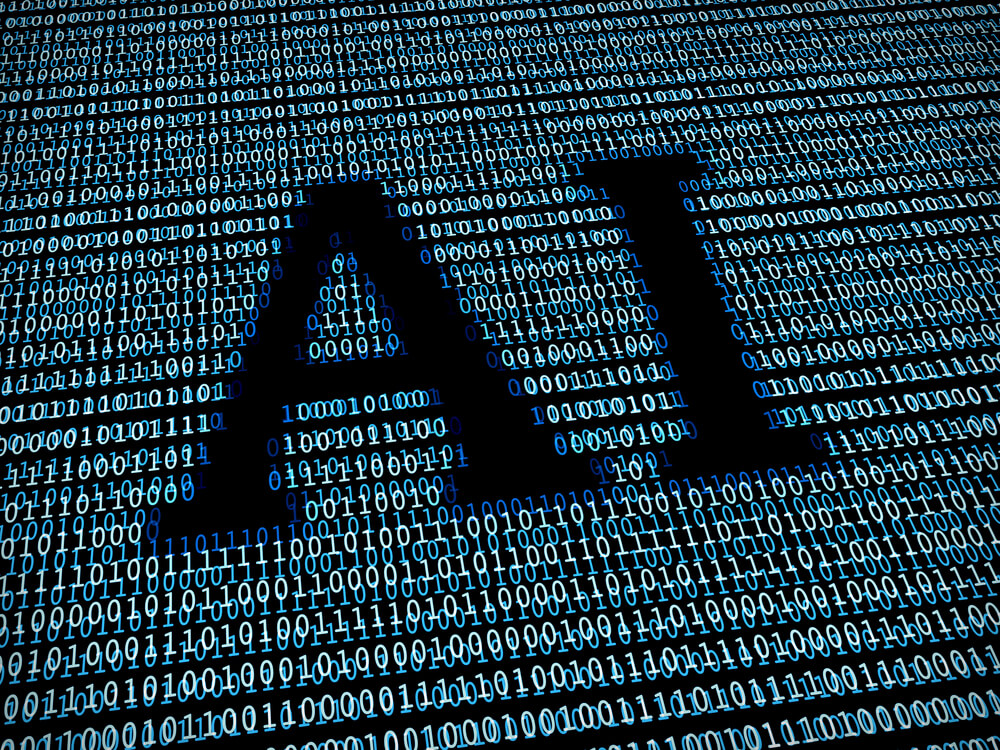
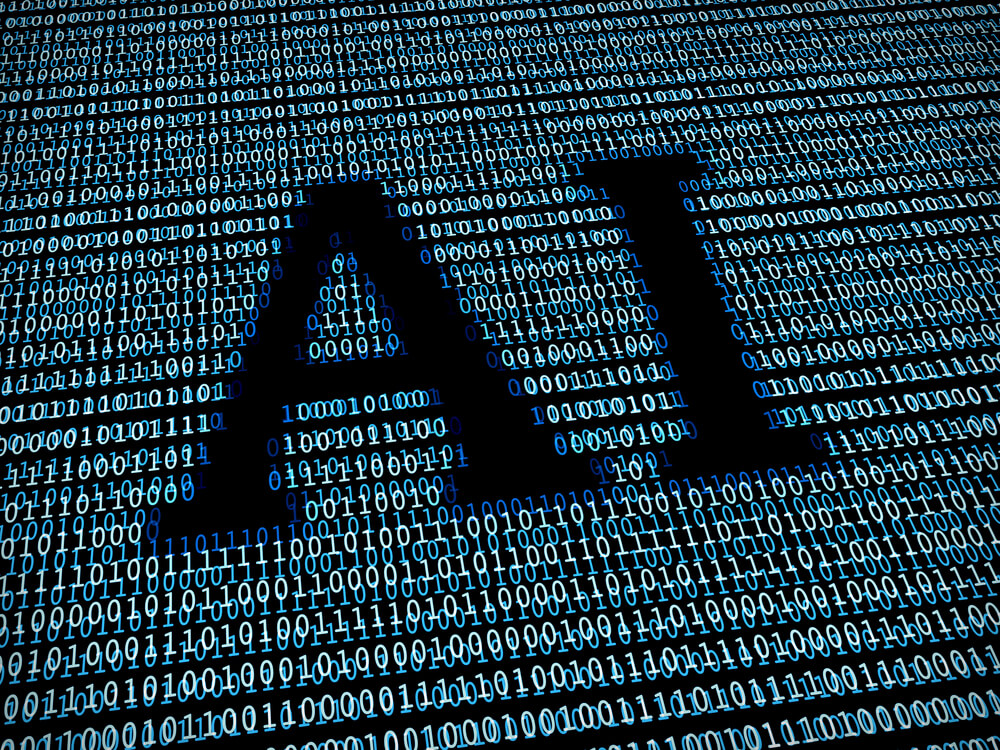
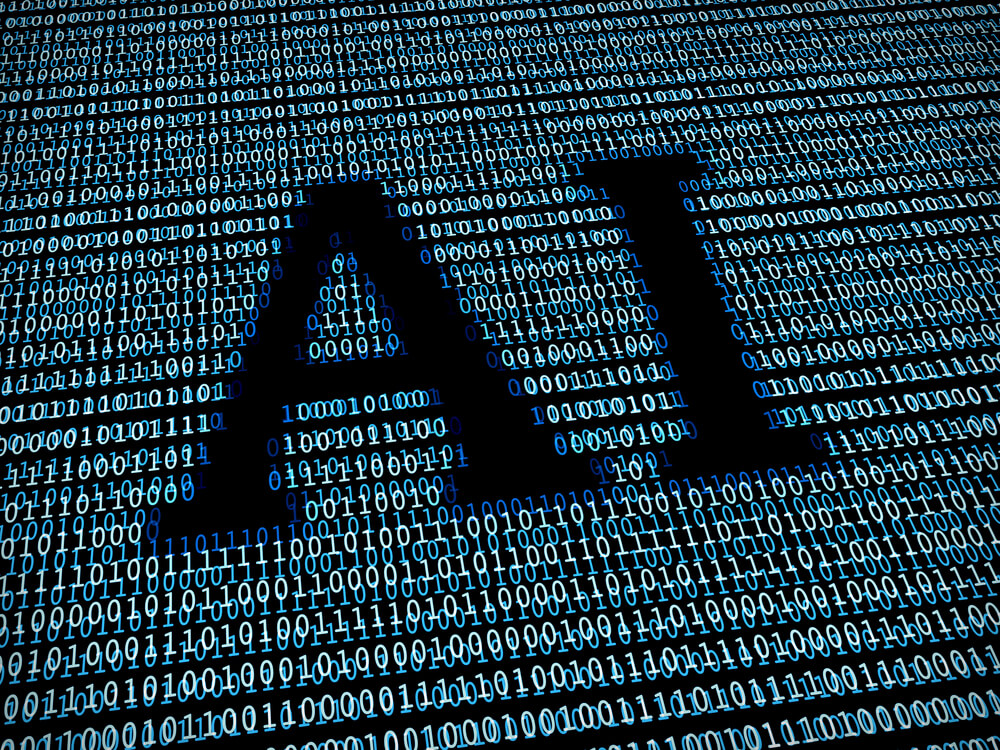
Get new exclusive access to healthcare business reports & breaking news
AI algorithms should meet certain requirements to combat bias in healthcare. This was the topic of discussion on December 1, 2020, at a session titled, “Artificial Intelligence and Implications for Health Equity: Will AI Improve Equity or Increase Disparities?” Four key healthcare professionals spoke during the event. Here is a snapshot of what they had to say on the topic.
Ziad Obermeyer, associate professor of health policy and management at the Berkeley (CA) School of Public Health, discussed something that he referred to as the pain gap phenomenon. This is the situation where caucasian patients suffering from any type of pain have that pain either treated or investigated until a cause can be identified and other races experiencing the same type of pain are either ignored or overlooked. He stated, “Society’s most disadvantaged, non-white, low income, lower educated patients…are reporting severe pain much more often.” He added, “An obvious explanation is that maybe they have a higher prevalence of painful conditions, but that doesn’t seem to be the whole story.” Obermayer stated that listening to the patient could be useful in finding the solutions to predicting the pain experience.
Luke Oakden-Rayner, director of medical imaging research at the Royal Adelaide (Australia) Hospital, offered an idea where exploratory error analysis could be used to look at every error case. The goal would be to identify common threads rather than taking a hard look at the AI model to try to pin down a bias. He explained the concept this way: “Look at the cases it got right and those it got wrong…all the cases AI got right will have something in common and so will the ones it got wrong, then you can find out what the system is biased toward.”
Constance Lehman, professor of radiology at Harvard Medical School, director of breast imaging, and co-director of the Avon Comprehensive Breast Evaluation Centre at Massachusetts General Hospital pointed out, “About two million women will be diagnosed with breast cancer and over 600,000 will die in the US this year…but there’s a marked discrepancy in the impact of breast cancer on women of color versus Caucasian women.” She developed an algorithm with another speaker at the event (Regina Barzilay, a professor in the department of electrical engineering and computer science and member of the Computer Science and AI Lab at the Massachusetts Institute of Technology). The algorithm assists in identifying women’s breast cancer risk based solely on a mammogram. Deep Learning (DL) is used along with an imaging coder that takes the four different views of a standard analog mammogram without needing to access family history, previous biopsies, or reproductive history. Lehman stated, “This imaging only model performs better than other models and supports equity across the races.” Barzilay added, “An image-based model that is trained on diverse populations can very accurately predict risk across different populations in a very consistent way.”
In the November 2020 Scientific American, an article explored the lack of diversity in medical data. Citing early clinical trials where women and minority groups were underrepresented as study participants, evidence started to surface that pointed to these groups as experiencing fewer benefits and increased amounts of side effects coming from approved medications. It became so obvious, that in 1993 a joint effort that included representation from the National Institute of Health (NIH), US Food and Drug Administration (FDA), researchers, industry members, and an act of Congress was launched. It continues to be a work in progress in 2020. To give you an idea of how important diversity in medical data has become, one company that has been developing a COVID-19 vaccine announced a delay until they were able to recruit more diverse participants in the clinical trial stage of development.
In many cases, the diversity of the AI algorithms represents just a tiny cross-section of the US population. For example, a study published in the Journal of American Medical Association revealed that when the diagnostic skills of doctors were tested against their AI counterparts across various aspects of clinical medicine, the digital results were poor. Well, that is to say, human doctors fared better. That was because the data that had been used to train the AI algorithms was comprised of medical data from just three states: New York, California, and Massachusetts. It doesn’t take a geography major to point out that this is just a small portion of the US population. The study proved that medical AI has a serious data diversity issue that shows in race, gender, and geography. What compounds the problem is that researchers are unable to access large pools of diverse medical data files and the result is clear – you end up with biased algorithms.
The sharing of medical data would be the best first step to correcting the current imbalance of information being used to train AI algorithms. However, privacy laws may restrict what can and cannot be shared. These hurdles need to be removed or some other way to gather data from a more diverse population will be required to eliminate the existing bias. As Regina Barzilay said, “Humans who are ultimately responsible for making a clinical decision should understand what the machine is doing, to think of all possible biases that the machine can introduce…models that can make their reasoning understandable to humans could help.”
AI algorithms are effective tools in assisting with healthcare applications. However, the medical data used to teach these algorithms have started showing bias based on the data that is used. By providing medical information that is more diverse can reduce this issue. However, access to medical records has posed another roadblock. The upside is that AI is with us and is going to continue to be with us well into the future. By working to remove the bias that has developed, AI can become a much more valuable tool in diagnostic treatment than it has been to date.