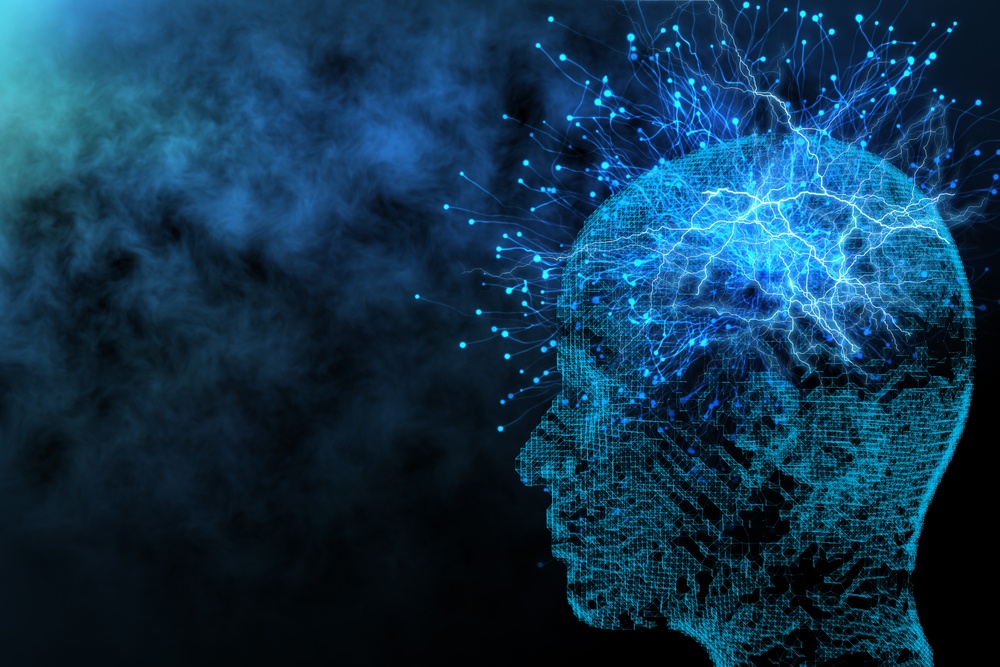
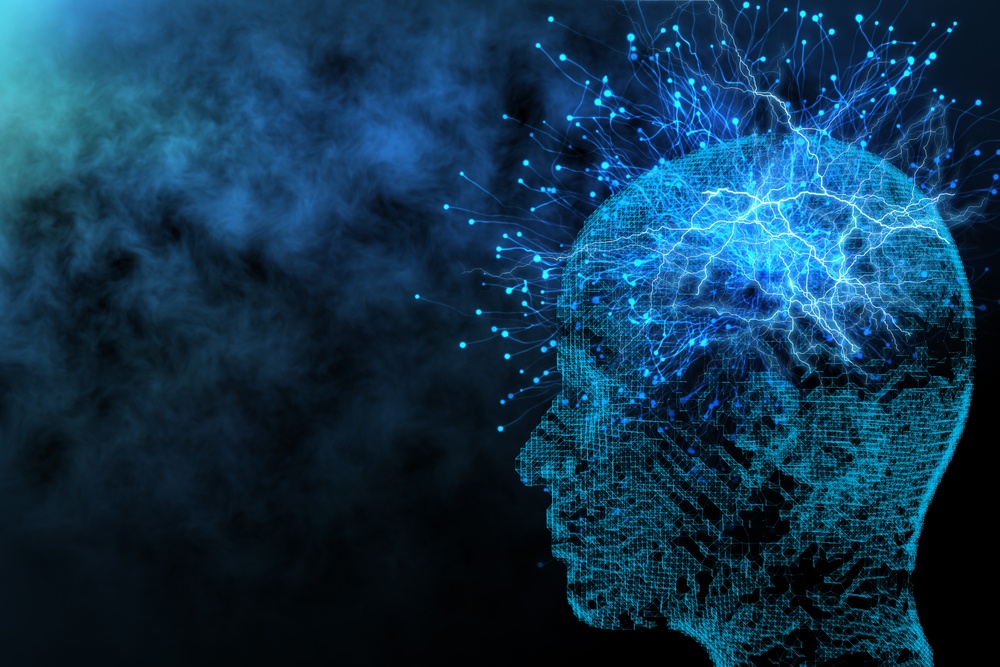
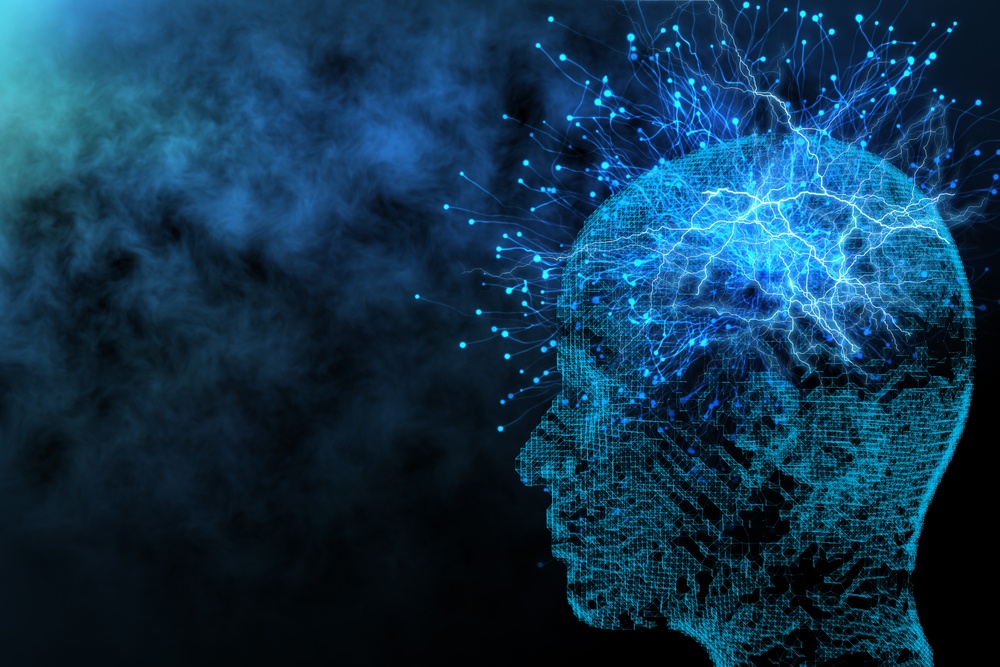
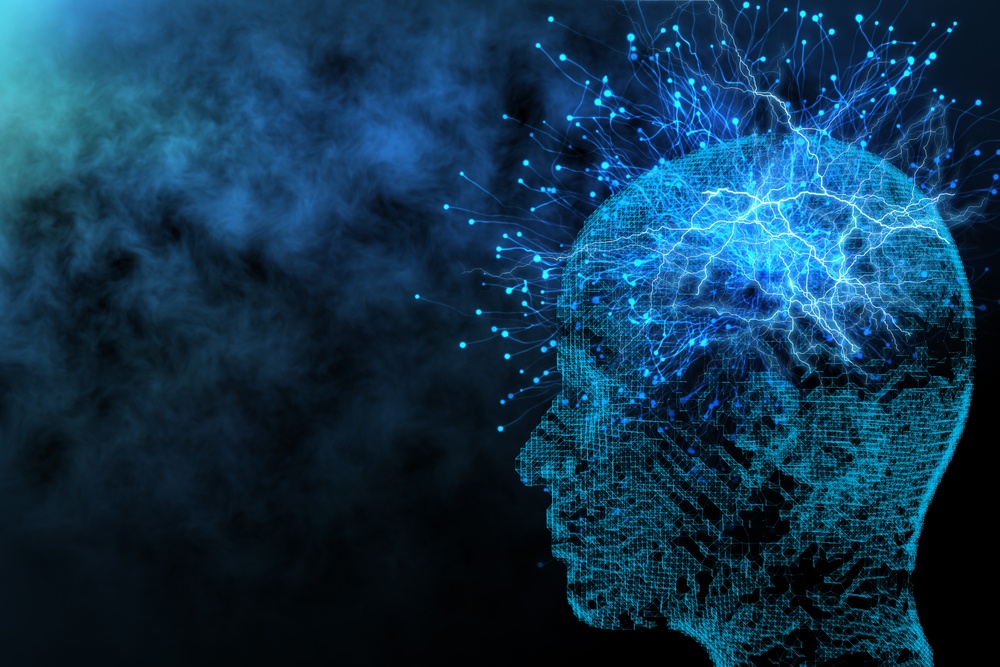
Get new exclusive access to healthcare business reports & breaking news
Around 44 million people in the world are living with either Alzheimer’s disease (AD) or a related form of dementia. In the U.S., AD is the sixth leading cause of death; an estimated 5.5 million people have the disease. The majority, around 5.3 million, are 65 and older; about 200,000 younger patients suffer from an early-onset type. According to the Alzheimer’s Association, this number is projected to rise to 14 million by 2050. In 2019, AD and other dementias will cost the nation $290 billion. By 2050, these costs could rise to as high as $1.1 trillion.
Although 82 percent of seniors in the United States consider having their thinking or memory checked important, only 16 percent say they receive regular cognitive testing.
Making diagnosis easier to establish is increasingly important, since more and more people are suffering from the disease, and many of the traditional memory assessment tools doctors have at their disposal are not sufficiently precise and reliable.
Researchers at Florida Atlantic University’s College of Engineering and Computer Science, SIVOTEC Analytics, HAPPYneuron, MemTrax, and Stanford University School of Medicine think artificial intelligence could be used for testing and managing Alzheimer’s, a complex human health condition.
Even in the case of MemTrax, an online memory test using image recognition, its clinical efficacy as a memory function screening tool has not been sufficiently demonstrated or validated. When interpreting memory evaluation test results, one has to consider various complex and interdependent factors, so the task becomes very difficult for clinicians. In order to address the growing number of AD cases, steps have to be taken to give doctors better tools to help them prevent, diagnose and better treat AD. Researchers employed a novel application of supervised machine learning and predictive modeling to demonstrate and validate the cross-sectional utility of MemTrax as a clinical decision support screening tool for assessing cognitive impairment.
Subsequently published in the Journal of Alzheimer’s Disease, the study introduces supervised machine learning as a modern complementary tool in cognitive brain health assessment and related patient care and disease management.
MemTrax results demonstrate its potential valid clinical utility when administered as part of the online Continuous Recognition Tasks (M-CRT) test, during screening for variations in cognitive brain health.
“Machine learning has an inherent capacity to reveal meaningful patterns and insights from a large, complex inter-dependent array of clinical determinants and the ability to continue to ‘learn’ from the ongoing utility of practical predictive models,” said Taghi Khoshgoftaar, Ph.D., co-author and Motorola Professor in FAU’s Department of Computer and Electrical Engineering and Computer Science. “Seamless use and real-time interpretation will enhance case management and patient care through innovative technology and practical and readily usable integrated clinical applications that could be developed into a hand-held device and app.”
For the study, researchers used an existing dataset (18,395) from HAPPYneuron. They examined answers to general health screening questions (addressing memory, sleep quality, medications, and medical conditions affecting thinking), demographic information, and test results from a sample of adults who took the MemTrax (M-CRT) test for episodic-memory screening.
“Findings from our study provide an important step in advancing the approach for clinically managing a very complex condition like Alzheimer’s disease,” said Michael F. Bergeron, Ph.D., senior author and senior vice president of development and applications, SIVOTEC Analytics. “By analyzing a wide array of attributes across multiple domains of the human system and functional behaviors of brain health, informed and strategically directed advanced data mining, supervised machine learning, and robust analytics can be integral, and in fact necessary, for health care providers to detect and anticipate further progression in this disease and myriad other aspects of cognitive impairment.”
In Florida, there are more than 540,000 people living with Alzheimer’s disease (AD), the most recognized form of dementia. The number of people in Florida who are age 65 and older with AD is expected to increase 41.2 percent by 2025 to a projected 720,000, which makes finding medical and treatment innovations a matter of great urgency.
In December of 2018, Florida Gov. Rick Scott announced research fellows from the Florida Atlantic University Brain Institute received a total of $1,137,227 from the Florida Department of Health’s Ed and Ethel Moore Alzheimer’s Disease Research Program. The program supports research leading to the prevention and possible cure for AD as well as better prevention, diagnosis, and treatment.
“Florida is No. 1 in per capita cases of Alzheimer’s disease in the United States,” said Randy D. Blakely, Ph.D., director of FAU’s Brain Institute. “Members of the FAU Brain Institute are addressing and tackling all aspects of Alzheimer’s disease from behavior to biomedicine and engineering. The funding support that we have received from the Florida Department of Health is a testament to the cutting-edge research in Alzheimer’s disease biology and interventions that is taking place throughout our university and will help us to continue to address a disease that takes a tremendous toll on patients, their families and society.”
Researchers from Florida Atlantic University’s Christine E. Lynn College of Nursing also conducted a study, published in the journal Aging & Mental Health , to test a new tool, the “Basic Knowledge of Alzheimer’s Disease” (BKAD). The study was conducted at senior centers in five rural communities in Florida (the Glades), Appalachian Virginia, West Virginia, Alabama and North Carolina. The aim was to measure and assess how much people in rural and underserved communities know about AD prevention, diagnosis and treatment.
Results showed three-fourths of the participants were aware annual cognitive screening is recommended for older adults, but only one-fourth had been previously screened. The majority of participants (86 percent) declared they would get tested annually, if offered the chance.
Finding a way to help clinicians better apply and interpret memory assessment tests is therefore immensely important, since this would ensure more people get tested more frequently, thus helping to prevent AD onset, and better manage the disease once it appears.
A separate study, published in Radiology, showed that, when machine learning (ML)-a type of artificial intelligence-is used with a regular heart scan, better results at predicting heart attacks and other cardiac events are achieved than with conventional risk models.
Heart disease is the leading cause of death for both men and women in the United States. Early intervention, such as diet, exercise and appropriate drugs can lower the risk considerably, so assessing said risk becomes crucial. Risk determination is, however, as imperfect as memory assessment tests in diagnosing Alzheimer’s disease. Popular existing models like the Framingham Risk Score are limited, since they do not take into account the condition of the coronary arteries.
Coronary computed tomography arteriography (CCTA) gives detailed images of the heart blood vessels, and are a promising tool for better risk assessment. Consequently, a multidisciplinary working group recently introduced a scoring system to `translate` CCTA results. Known as the coronary artery disease reporting and data system (CAD-RADS), the decision-making tool focuses on coronary stenosis–blockages or narrowing in the heart arteries.
The lead author of this recent study, Kevin M. Johnson, M.D., associate professor of radiology and biomedical imaging at the Yale School of Medicine in New Haven, Conn, noted that, although CAD-RADS is useful in the management of cardiac patients, its focus on stenosis may leave out other decisive data about the arteries.
CCTA reveals more than just stenoses, so Dr. Johnson investigated an ML system able to trap the myriad details in these images, and use them to create a more comprehensive prognostic picture.
“Starting from the ground up, I took imaging features from the coronary CT,” he said. “Each patient had 64 of these features and I fed them into a machine learning algorithm. The algorithm is able to pull out the patterns in the data and predict that patients with certain patterns are more likely to have an adverse event like a heart attack than patients with other patterns.”
Dr. Johnson and his colleagues compared the ML approach with CAD-RADS and other vessel scoring systems in 6,892 patients. After they underwent a CCTA, patients were followed for nine years, on average. 380 of them died from all causes, 70 of those were from coronary artery disease. 43 patients reported they suffered heart attacks.
When compared to CAD-RADS and other scores, the ML solutions better selected patients more at risk for a cardiac event, distinguishing them from those who would not suffer one. Even more impressive, the decision to start patients on statins was easier to reach with the aid of AI. The ML score ensured that 93 percent of patients with events would receive the drug, compared with only 69 percent if CAD-RADS were relied on.
“The risk estimate that you get from doing the machine learning version of the model is more accurate than the risk estimate you’re going to get if you rely on CAD-RADS,” Dr. Johnson said. “Both methods perform better than just using the Framingham risk estimate. This shows the value of looking at the coronary arteries to better estimate people’s risk.”