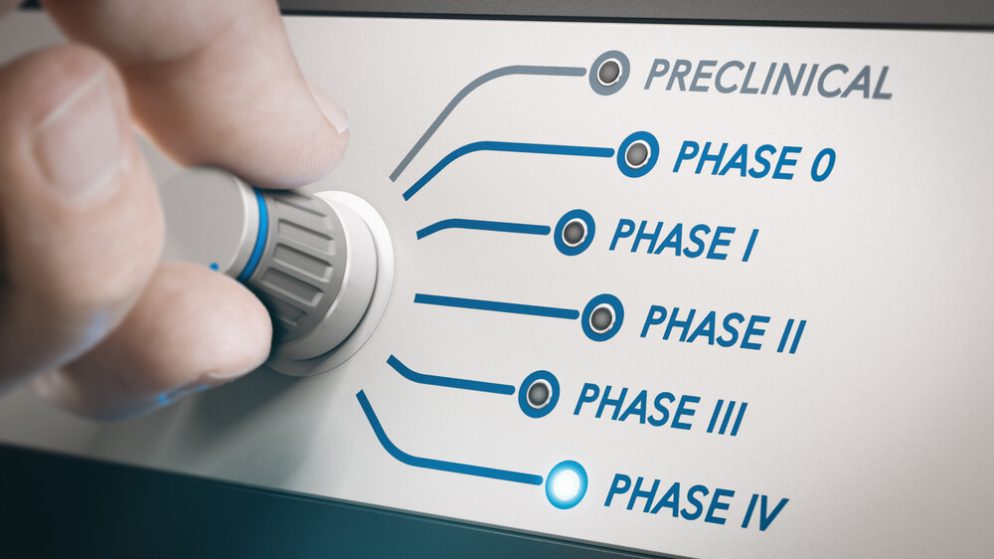
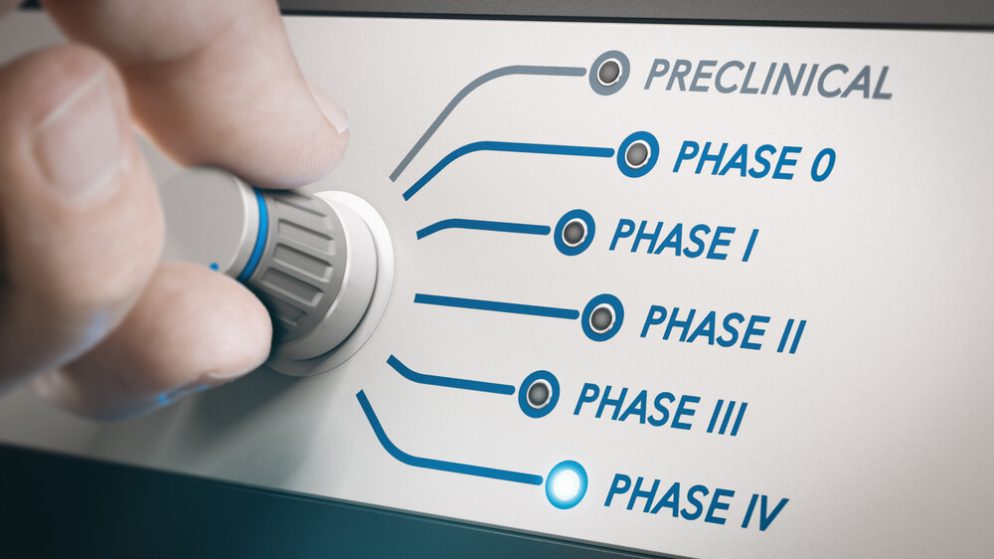
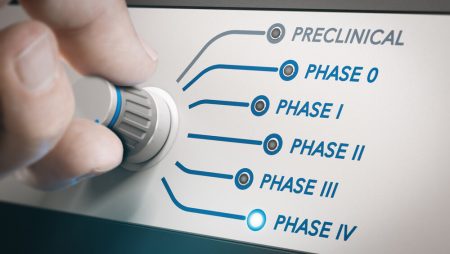
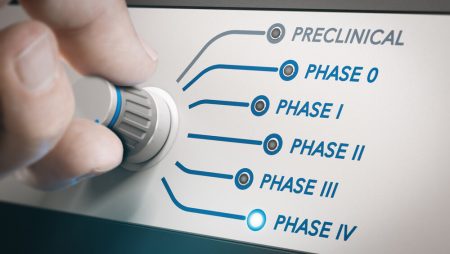
Get new exclusive access to healthcare business reports & breaking news
Clinical trials are essential to medical research, allowing scientists and medical professionals to evaluate the safety and effectiveness of new treatments and interventions. These trials involve human participants and follow a specific design to ensure accurate and reliable results. This article will delve into the best practices for dataset design in clinical trials, focusing on using Define-XML.
Effective dataset design is crucial for the success of clinical trials. It involves defining the structure and layout of data collected during the trial ensuring that the information captured is accurate, reliable, and compliant with regulatory standards. A well-designed dataset facilitates smooth data collection, analysis, and interpretation, leading to credible and reproducible outcomes.
Define-XML for dataset design is an industry-standard data exchange format used in clinical trials to define and communicate the structure and contents of datasets. It provides a standardized framework for describing data elements, their relationships, and associated metadata. Define-XML allows for accurate and consistent interpretation of clinical trial datasets, enhancing stakeholder collaboration and interoperability.
It is important to adhere to certain best practices to optimize dataset design in clinical trials. By following these guidelines, researchers can ensure the quality and integrity of their data while promoting efficiency and transparency in the trial process. Some key best practices include:
Standardizing the dataset structure and variables across trials allows for easier data integration and comparison. By adopting standardized data collection instruments, consistency is ensured, enabling the pooling of data for meta-analyses and promoting interoperability among different studies.
Provide clear and concise definitions for each variable in the dataset. These definitions should be unambiguous and easily understandable by all users involved in the trial. Well-defined variables promote accurate data collection and interpretation, reducing the likelihood of errors and inconsistencies.
Establish coding and formatting conventions for variables in the dataset. This involves specifying acceptable values and formats for each variable, ensuring consistency throughout the dataset. Consistent coding and formatting minimize errors during data entry and analysis, enhancing data quality and facilitating data management processes.
Maintain documentation of dataset versions, including updates and revisions. Documenting changes in the dataset ensures transparency and traceability, allowing all users to understand the chronological evolution of the dataset over time. Proper versioning and documentation practices facilitate collaboration and data reuse in subsequent studies or analyses.
Define relationships and linkages between variables in the dataset. Establishing these connections enables efficient data analysis and helps identify inconsistencies or missing data. By understanding the relationships between variables, researchers can identify potential data quality issues and conduct more accurate analyses.
Data quality and integrity are paramount in clinical trials. To maintain these standards, datasets must be designed with robust measures. Some considerations for ensuring data quality and integrity include:
It is important to implement data validation checks to ensure that the data entered into the dataset meets predefined criteria. These checks can include simple validations like range and data type checks and more complex ones like logic and cross-field validations. By validating the data, researchers can identify and prevent any inaccuracies or inconsistencies in the dataset.
Regular monitoring of data collection processes is necessary to promptly identify and address any issues that may arise. This monitoring can involve conducting data audits to assess the completeness and accuracy of the data. If any discrepancies are identified, corrective actions can be implemented to rectify the problem and ensure the accuracy and reliability of the dataset.
Protecting the confidentiality and privacy of trial participants’ data is essential. Proper security measures should be implemented to safeguard sensitive information from unauthorized access. This may involve employing techniques such as encryption, access controls, and data anonymization to ensure that participant data remains confidential and secure throughout the trial.
Accurate statistical analysis is fundamental to drawing meaningful conclusions from clinical trial data. Proper dataset design helps ensure that the collected data can be analyzed effectively. Some key considerations for statistical analysis in dataset design include:
Properly coding and formatting variables is crucial for effective statistical analysis. Categorical variables should be assigned numerical codes to enable quantitative analysis. Data should also be organized in a format suitable for statistical software, ensuring variables are correctly labeled and structured.
Missing data can introduce bias in statistical analyses, so it is important to establish protocols for handling these missing values. Consider different approaches, such as imputation methods, where missing data points are replaced with estimated values based on patterns in the existing data. Sensitivity analyses can also be performed to evaluate the impact of missing data on the results.
Outliers and extreme values can significantly impact statistical analyses. It is essential to develop strategies for identifying and handling these data points. A careful assessment should be made to determine whether outliers are valid data points or errors. Depending on the nature of the data and the analysis goals, appropriate actions can be taken, such as removing outliers, transforming variables, or conducting separate analyses with and without outliers.
Sharing clinical trial data promotes scientific progress, collaboration, and transparency. Dataset design should consider data-sharing requirements and compliance with relevant regulations and guidelines. Some considerations for data sharing and compliance include:
Anonymize participant data to protect privacy when sharing datasets. Remove or modify identifying information to ensure that individuals cannot be re-identified from the data.
Ensure the dataset design adheres to relevant regulatory standards, such as those set by the Food and Drug Administration (FDA) or the International Council for Harmonization of Technical Requirements for Pharmaceuticals (ICH). Compliance with these standards ensures the dataset’s acceptance and compatibility across different studies.
Dataset design plays a vital role in the success of clinical trials. Adhering to best practices, such as standardization, clear variable definitions, and consistent coding, ensures accurate and reliable data collection. Robust measures for data quality, statistical analysis, and compliance with regulatory standards further enhance the value and integrity of clinical trial datasets. By following these best practices, researchers can effectively design datasets that contribute to accurate and meaningful outcomes in clinical research.