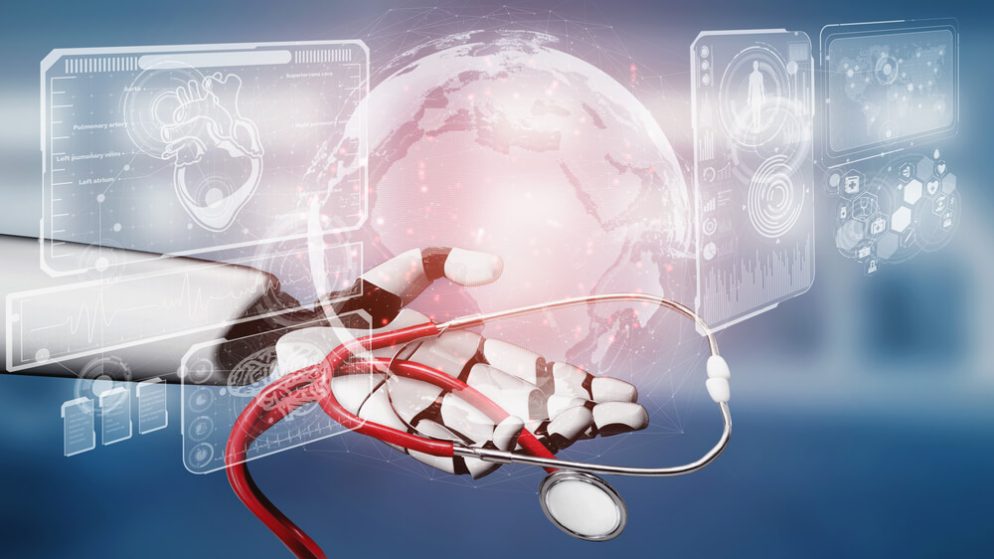
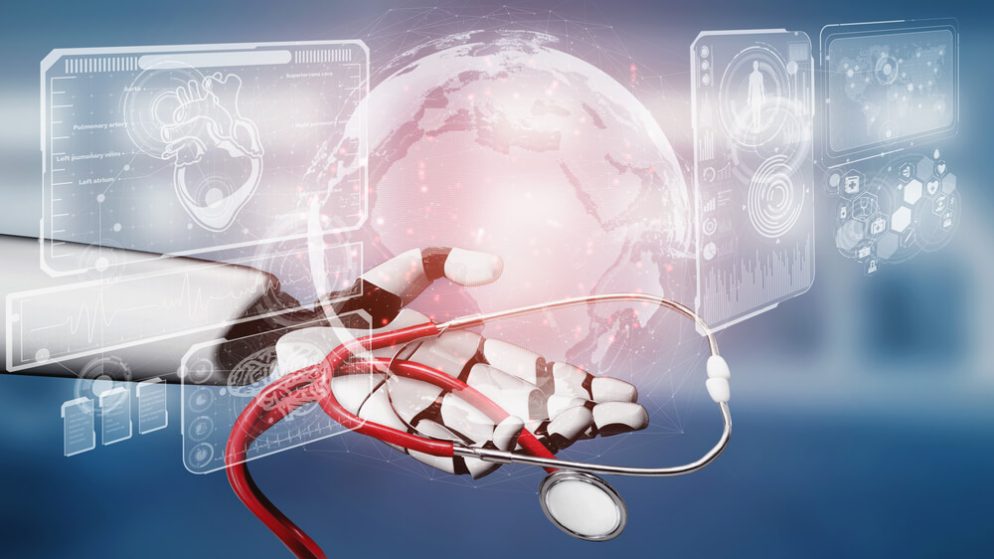
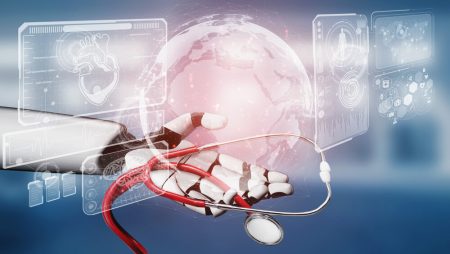
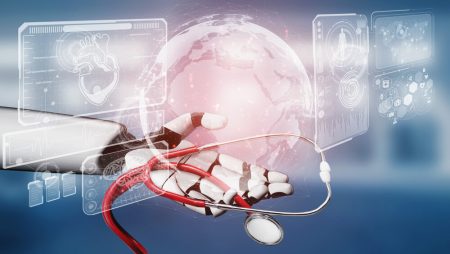
Get new exclusive access to healthcare business reports & breaking news
By Dr. Amanda Randles
In 2006, British mathematician Clive Humby proclaimed: “Data is the new oil.” The analogy expresses the great value that data holds in the modern world and the critical role it plays in fueling a wide range of industries. It also speaks to the fact that data, like oil, must be processed before its value can be realized.
Converting raw data to applicable insights is a labor-intensive and resource-intensive task. The volume of data that is collected daily in most industries is overwhelming. Statistics show that 95 percent of businesses struggle with efficiently managing their unstructured data, let alone analyzing it.
Those who are effectively mining data have found machine learning to be an invaluable tool. Machine learning is a type of artificial intelligence that streamlines the data analysis process by allowing computers to build analytical models automatically. With machine learning, computers move beyond compiling and presenting to data to learning from the data. This learning allows them to identify patterns and make data-based decisions independently — and at a much faster pace than human analysis allows.
In the healthcare industry, machine learning promises to increase the quality of care that patients can receive dramatically. Modern healthcare produces a staggering amount of data. Some findings show that as much as 30 percent of the world’s data output is healthcare-related. Insights extracted from that data can empower a wide range of healthcare enhancements, from better patient management to more streamlined diagnoses, more effective responses, and emerging epidemics.
Here are a few examples of emerging applications that reveal the promise machine learning holds for the future of healthcare.
Medical diagnosis primarily involves extracting meaning from medical information. Medical professionals review a patient’s vital signs, past medical history, and symptoms to identify conditions and prescribe a course of action. In some situations, diagnosis requires analyzing a considerable amount of data. With patients facing severe health risks, decreasing the time needed for diagnosis and treatment can often lead to better results.
For example, for a patient presenting with symptoms of cardiac disease, the doctors need to determine if a blockage exists and if the severity of that blockage necessitates intervention. ML is increasingly playing a role in medical image analysis and reducing the time required to analyze images and identify blockages. The combination of ML with physics-based models of a patient’s blood flow further reduces our reliance on invasive procedures and allows faster assessment of lesion severity.
We are also developing new ML-driven pipelines for providing intervention guidance. Coronary bifurcation lesions provide an example of a condition that benefits from this application. These lesions occur when the narrowing of a coronary artery also affects an adjacent side branch. To effectively treat these lesions, doctors must determine where to position a stent based on how they believe the lesion will develop. Machine learning is being applied to empower predictive models that determine how the lesion is most likely to develop for each unique patient. By utilizing these models, doctors can quickly and efficiently determine the best course of treatment.
With medical conditions developing in a patient, doctors must prescribe action based on how those conditions typically evolve. As more data on such conditions is gathered and analyzed, it becomes easier to predict what could occur and what treatment will be most effective. Machine learning is a powerful tool for analyzing data and making predictions on a patient-by-patient basis.
To understand how a condition will evolve, blood flow must be assessed over various circumstances, such as while resting and exercising or, even more complex, running at altitude. Creating virtual representations of a patient’s blood flow allows doctors to assess how a patient’s blood flow would change under real-world conditions where invasive measurement would not be feasible. This innovation significantly streamlines the data-gathering process but still requires massive computing power to run the necessary simulations. By applying machine learning to analysis, the time needed for running simulations has been reduced from approximately 70 million compute hours per patient to approximately 4 million hours, marking a 94 percent improvement. With ongoing work, we expect to see even further reduction, making such prospective modeling tractable.
Machine learning holds promise for the medical industry on the administrative and clinical side. The shift to electronic health records (EHR) has resulted in mountains of data being collected by healthcare providers. Machine learning empowers the degree of accuracy and speed that is necessary to mine EHR data for insights. Whether the task involves identifying inefficiencies in billing procedures or alerting doctors when patients are not responding as predicted to prescribed treatments, machine learning holds the potential to improve the systems that support the modern healthcare process dramatically.
By some estimates, as much as 80 percent of the data that the medical industry gathers remains unstructured. In other words, there is a wealth of untapped information available to medical professionals that could provide invaluable insights. Leveraging machine learning to mine this data promises to dramatically improve the efficiency and effectiveness of the entire healthcare arena.